These researchers made AI consume 95% less energy. You just need to multiply better
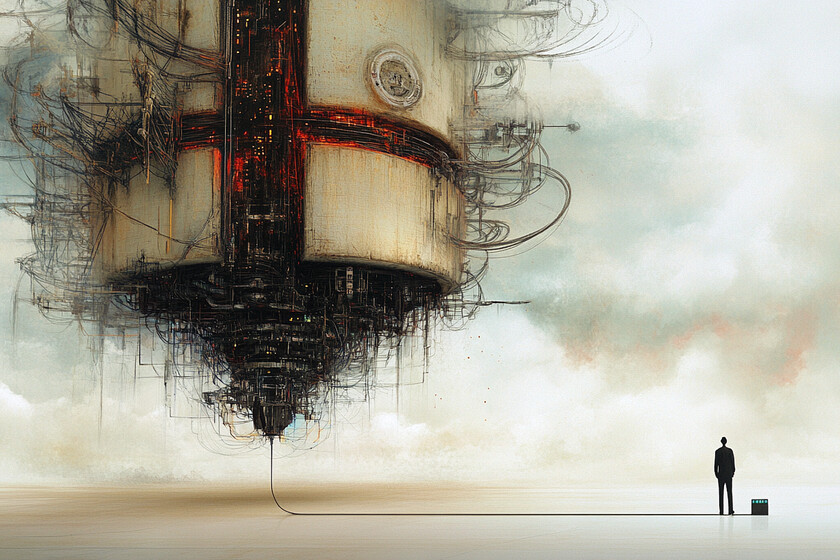
Every time we use ChatGPT or Midjourney, we contribute to an exceptional consumption of energy (and water!) This is one of the problems associated with the development of artificial intelligence, and companies are already thinking about using nuclear power plants to meet this demand. While many are eager to come up with methods to solve these energy needs, others are taking the exact opposite route: ensuring that AI consumes much less.
AI on a diet. As Decrypt notes, researchers from BitEnergy AI have developed a new technique for reducing energy consumption using these models. They estimate that their method can save up to 95% of energy consumption without compromising the performance of these systems.
Multiply better. The key to this diet is the way it multiplies floating point numbers, an operation that requires intensive AI calculations. Instead of multiplication, the researchers use integer addition, which, according to their analysis, can significantly reduce the energy consumption of the operation.
Numbers with commas. Floating point is a mathematical concept that allows computers to efficiently manipulate large and very small numbers using a simple operation: placing a decimal point (or, for countries with other notations, a period). The larger the number (“width”) of bits, the greater the accuracy we get in the calculations, but also the greater the energy cost (or system memory requirements). Therefore, for example, FP32 (used in deep learning algorithms) provides greater accuracy than FP8 (used in training and inference where such accuracy is not required).
L-Mule. The algorithm developed by these researchers replaces multiplication with addition of integers. It decomposes these multiplications using additions, which speeds up calculations and reduces power consumption without affecting the accuracy of the result.
Special equipment required. However, using this method has the disadvantage that it requires a specific type of hardware, and existing systems are not optimized to achieve this reduction. Despite this, the researchers claim that their algorithms are implemented using hardware chips, and this option is expected to become practically available in the near future.
Promising. The developers of the method claim that this method allows artificial intelligence systems to “potentially reduce the energy cost of multiplying floating point tensors by elements by 95% and dot products by 80%.” Tensors are multidimensional arrays of numbers that represent data in neural networks.
Matrix multiplication is a difficult task. Finding the best ways to multiply matrices has become a unique mathematical challenge for all types of businesses and companies. DeepMind has already unveiled its own system for improving matrix multiplication at the end of 2022, and just a year later, a team from the Polytechnic University of Valencia also proposed an equally promising alternative.
In Hatak | Physicists have something incredible: a very precise method for simulating black holes in their laboratory.