Artificial intelligence may help predict when cancer will resist chemotherapy
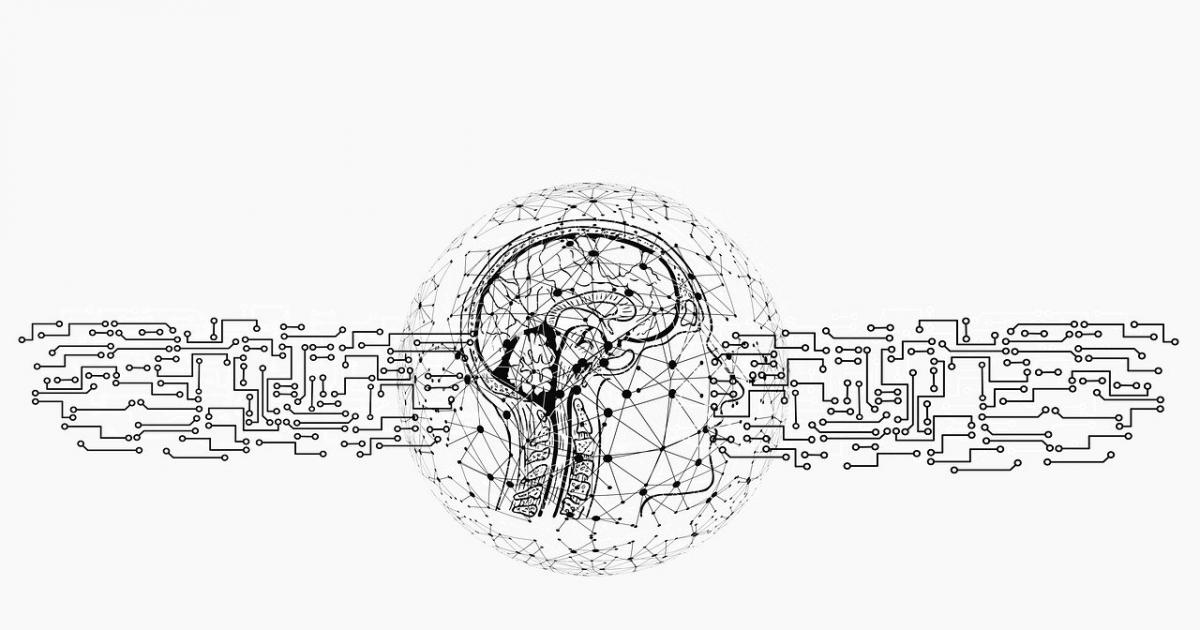
The journal Cancer Discovery publishes new work from the University of California San Diego School of Medicine (USA), according to which it was shown that the machine learning algorithm achieves predict when cancer will resist chemotherapy.
To understand how this works, the researchers emphasize that all cells, including cancer cells, depend on the complex molecular machinery of DNA replication as part of normal cell division. Most chemotherapy drugs work by disrupting this DNA replication machinery in rapidly dividing tumor cells.
Although scientists recognize that a tumor’s genetic makeup greatly influences its specific response to drugs, the large number of mutations found in tumors has made predicting drug resistance a challenging task.
New algorithm overcome this barrier by examining how multiple genetic mutations collectively influence how tumors respond to drugs that prevent DNA replication.
In particular, they tested their model on cervical cancer tumors and they successfully predicted responses to cisplatin, one of the most common chemotherapy drugs. The algorithm was able to identify tumors with the highest risk of treatment resistance, as well as identify most of the underlying molecular mechanisms that cause treatment resistance.
“Previously, doctors were aware of some isolated mutations that were associated with treatment resistance, but these isolated mutations generally did not have significant prognostic value. The reason is that a much larger number of mutations can shape the response to cancer treatment. That used to be the case,” said Trey Ideker, a professor at the University of California San Diego School of Medicine.
“Artificial intelligence closes this gap in our understanding, allowing us analyze a complex range of thousands of mutations simultaneously“, he notes.
The researchers focused on a standard set of 718 genes commonly used in clinical genetic tests to classify cancer, using mutations in these genes as input to their machine learning model.
After training on publicly available drug response data, the new algorithm identified 41 molecular clusters (groups of helper proteins) in which genetic changes influence drug effectiveness.
Moreover, in addition to predicting response to treatment, this model helped shed light on the decision-making process when identify sets of proteins that cause treatment resistance for cervical cancer. The researchers emphasize that this aspect (the ability to interpret its reasoning) is key to the success of the model, as well as to the creation of reliable artificial intelligence systems.
“The researchers therefore conclude that they are optimistic that this model will have broad applications not only for improving existing cancer treatments, but also for creating new ones.”