Google’s artificial intelligence brings us closer to understanding the cellular universe
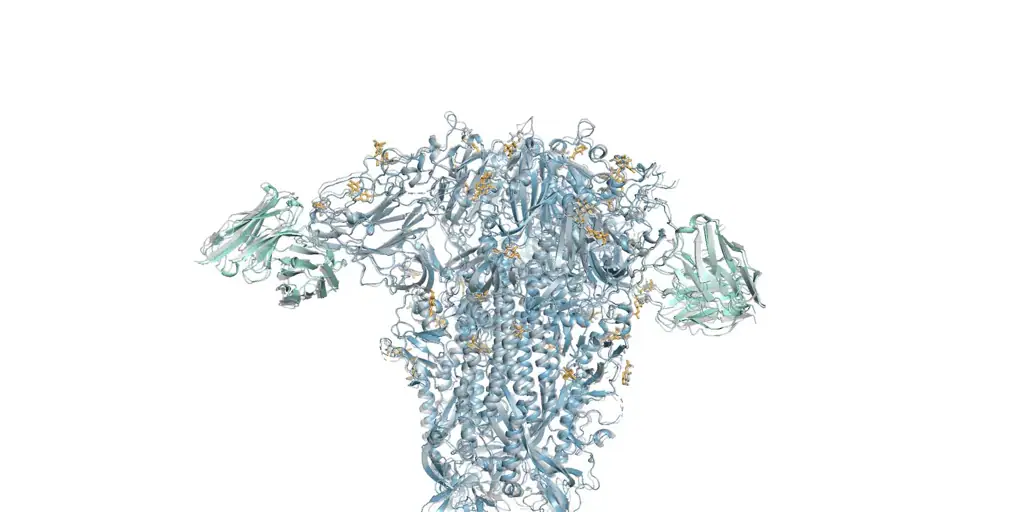
Inside cells exists a hidden universe as fascinating and vast as outer space. There, biological molecules, mainly proteins and nucleic acids such as DNA and RNA, orchestrate a complex symphony of interactions and chemical reactions that create … life is possible. Understanding how this microscopic universe works is essential to advancing disease treatments and enabling biotechnological advances.
The branch of science that studies the complexity of the internal structure of a cell in atomic detail is called structural biology. The discipline emerged in the mid-20th century with the creation of DNA double helix structures and the first proteins (hemoglobin and myoglobin), two milestones in biological research recognized by two Nobel Prizes. It will take years and the use of advanced technology to apply this experimental approach, which has determined the structure of about 200,000 proteins, representing about one-thousandth of the protein universe.
In 2020, DeepMind, a subsidiary of Google, developed an artificial intelligence known as AlphaFold, which, after learning from experimentally obtained protein structures, was able to predict the structure of any other protein with high confidence. Two years later, DeepMind and the pan-European research center EMBL launched a publicly accessible database with AlphaFold 2 predictions for all known proteins—about 200 million.
Published this Wednesday in the journal Nature, Google’s AI enhancement, AlphaFold 3, represents an important step forward for several reasons. First of all, because it can predict the structures of not only proteins, but also nucleic acids. Secondly, because it can reveal the structure of proteins with chemical modifications necessary for their functionality. And third, because it predicts interactions between proteins and other smaller molecules that regulate their activity.
This latest development could revolutionize drug discovery. Drug discovery is a complex and expensive task, which in the initial stages involves the chemical synthesis of a large number of compounds and their experimental testing on animals. AlphaFold 3 demonstrated significant improvement over other tools, which should significantly reduce development and animal testing time.
Despite its potential, AlphaFold 3 is not without limitations, as the authors note. For example, in complex cases, the algorithm predicts regions of different molecules occupying the same space, which is impossible. For flexible regions of proteins that lack an ordered shape, they can be predicted to adopt a specific structure, which can lead to erroneous interpretations. The algorithm also does not accurately predict the dynamism of molecules required for their function. Finally, the authors provide a limited number of success stories.
On the other hand, it is surprising that the code is not open and the method is offered on a server “with restrictions on allowed ligands and covalent modifications,” as the publication notes. It is hoped that public and open initiatives, such as the one led by David Baker at the University of Washington, will develop similar tools. In conclusion, innovations in artificial intelligence applied to biology, coupled with experimental studies that provide meaning and complementary predictions, predict a new era in understanding the microscopic universe that inhabits cells.